Study watch: Risky Artificial Intelligence
- Harry Law
- Jun 9, 2023
- 7 min read
A review of 'Risky Artificial Intelligence: The Role of Incidents in the Path to AI Regulation' by Giampiero Lupo of the Institute of Applied Sciences and Intelligent Systems, National Research Council of Italy.
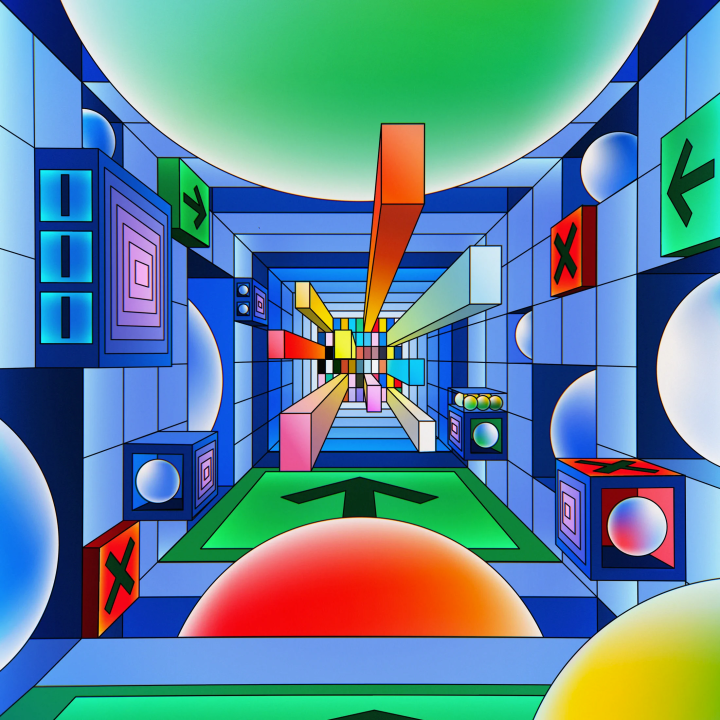
Champ Panupong Techawongthawon, Visualising AI by DeepMind
Earlier this week, Giampiero Lupo of the Institute of Applied Sciences and Intelligent Systems, National Research Council of Italy wrote in the Law, Technology and Humans journal on the role 'incidents' in shaping AI regulation. Lupo draws comparisons between AI and other new, complex technologies––such as the advent of nuclear power and the introduction of the automobile––to connect the cataloguing and analysis of AI incidents (defined as a situation in which AI systems caused, or nearly caused, real-world harm) with an ability to effectively regulate artificial intelligence.
The paper suggests that the spread of advanced technology tends to result in a divide between those excited by its novelty and those wary of its risks, while the coexistence of new and old technologies typically introduces novel challenges that require regulation in order to safely integrate them into society. It reminds us that, like other advanced technologies, concerns about safety, novel risks, and sudden changes disrupt the systems, structures, and arrangements that people are accustomed to and comfortable with.
On the one hand, norms can control technological change, restricting its scope and impact on the existing order; while on other, it is the sense of displacement that new technology brings that prompts a rush to regulate to restore or protect normalcy. This dynamic is particularly important "because AI refers to autonomous entities by definition and involves technologies that can act as intelligent agents that receive perceptions from the external environment and perform actions autonomously." As the paper later acknowledges, however, 'AI' is challenging to define: the technology exists as a constellation of data, people, power, and technological practice. AI systems might be agentic or tool-based, use-case specific or generalist, follow hard-coded rules or 'learn' from examples. Many of today's systems are not capable of 'autonomous' action, even fewer when using a restrictive definition. (Autonomy should not, however, be confused with capability or the potential to cause harm).
According to the paper, the regulation of emerging technologies (including AI) can be connected with two drivers: uncertainty about the potential impact of a technology and its risks, and information gathering in the aftermath of undesirable or unintentional events whose happening resulting in harm, injury, or damage. The latter, which is the primary focus of the author, are referred to as ‘incidents’. Incidents shape the regulation of emerging technologies because as technology becomes more sophisticated, it becomes harder to identify safety issues and their impact on individuals, society, and the environment in advance of their use.
Historical parallels: from automotive to AI
The theoretical basis through which AI incidents are connected with regulation is the notion that crises provide insight into the operation and effects of new technology. Drawing on Yale sociologist Charles Perrow's Normal Accident Theory, which suggests that situations exist wherein the complexity of systems unavoidably leads to accidents, the paper proposes that the sophistication of AI makes incidents both inevitable and unpredictable. In this framing, it is only after a crisis that safety issues can be identified, the technology's impact fully understood, and its inner workings revealed. Describing such moments as 'crises' is perhaps particularly apt when using the clinical definition: 'the turning point of a disease when an important change takes place, indicating either recovery or death.' Perrow's idea, which was originally formulated in the wake of the Three Mile Island disaster, is applied by the author to the automobile industry in the United States.
High risks and numerous casualties also had an impact on regulatory regimes that tried to improve the safety of the use of automotive technology. For instance, the 50,000 lives lost per year in 1966 in the United States contributed to the change of paradigm from ‘auto-safety’ to ‘crashworthiness’. The auto-safety paradigm was based on the assumption that as soon as nobody hits each other, no one will get hurt; therefore, this approach focuses on the ‘three Es’: (1) engineering roads to limit the possibility of collisions and equipping vehicles with reliable brakes and steering, (2) educating drivers and pedestrians to avoid collisions and (3) drafting and enforcing rules of the road to discipline drivers’ behaviour. In contrast, the ‘crashworthiness’ paradigm diffused since the late 1960s considered that a number of incidents on the road are unavoidable; therefore, car manufacturers had to design and implement technologies like seat belts and airbags that limit the impact of incidents on the human body.